Research on MRI reconstruction algorithm based on deep learning
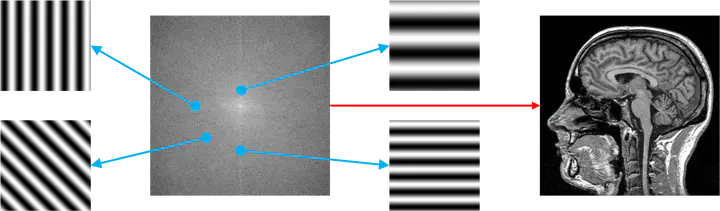
Magnetic Resonance Imaging (MRI) is a widely used diagnostic tool in clinical practice due to its numerous advantages, such as being non-invasive, free from ionizing radiation, and offering multi-parameter capabilities. However, MRI examinations are relatively slow, primarily because the original MRI data is acquired sequentially in k-space, and the traversal speed of k-space is limited by physiological and hardware constraints. This results in slower examination times compared to other imaging modalities. Prolonged scan times not only limit the efficiency of MRI devices but also increase patient discomfort and the risk of motion artifacts. Moreover, cardiac cine MRI, which demands higher imaging speed and motion artifact correction, presents additional challenges, especially when imaging under free breathing conditions, where more motion artifacts are inevitably introduced.
With the development of deep learning, this approach has made significant progress in fields such as computer vision and medical image processing. Simultaneously, deep learning has been successfully applied to MRI reconstruction tasks. Compared to traditional methods based on Compressed Sensing (CS), deep learning-based MRI reconstruction methods have certain advantages. Particularly in cardiac cine MRI reconstruction, deep learning-based methods can better correct motion artifacts. While past deep learning-based MRI reconstruction methods have shown some development, they haven’t fully leveraged multi-domain information for static MRI reconstruction. There is also room for further exploration of the model’s ability to correct motion artifacts in the context of cardiac cine MRI. Therefore, this paper focuses on exploring multi-domain information in MRI reconstruction and enhancing the correction of artifacts in the Cardiac Cine MRI reconstruction process.